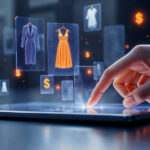
The Rise of Shoppable Programmatic Ads: Bridging Content and Commerce
April 16, 2025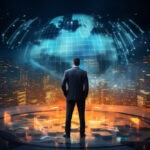
Cross-Device Targeting in Programmatic: Creating Seamless Consumer Journeys
April 18, 2025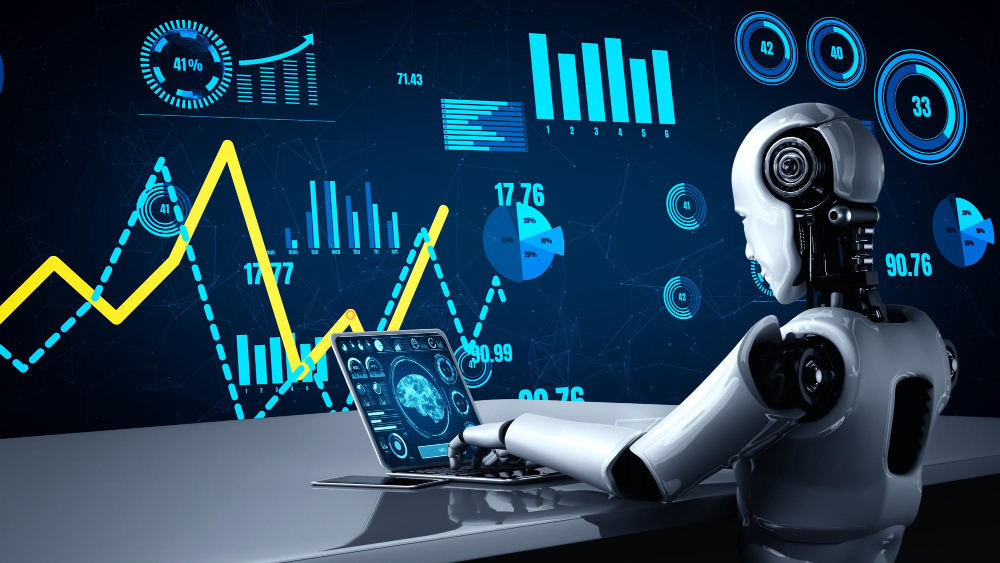
Leveraging Machine Learning for Predictive Budget Allocation in Programmatic
Introduction
In the fast-evolving world of digital marketing, programmatic advertising has become the cornerstone for brands seeking precise targeting, real-time bidding, and efficient media spend. Yet, one of the most challenging aspects of programmatic campaigns remains budget allocation—deciding how much to spend, where, and when to maximize return on investment (ROI).
Enter machine learning (ML)—a powerful technology that’s revolutionizing budget management by predicting the most effective allocation of resources across channels, audiences, and timeframes. This blog explores how machine learning enhances predictive budget allocation in programmatic advertising, helping marketers achieve smarter spend decisions and better campaign outcomes.
What Is Predictive Budget Allocation in Programmatic Advertising?
Predictive budget allocation refers to using historical data and advanced algorithms to forecast where advertising dollars will deliver the highest impact. Instead of relying on manual intuition or static rules, machine learning models analyze vast datasets to identify patterns and optimize budgets dynamically.
In programmatic advertising, this means ML-driven systems can:
Predict which audiences are likely to convert at different times
Allocate spend efficiently across platforms like display, video, Connected TV (CTV), audio, and social
Adjust bids in real time to balance cost and value
Forecast campaign performance before launching, enabling proactive planning
Why Traditional Budget Allocation Falls Short
Traditionally, marketers allocate budgets based on past campaign results, industry benchmarks, or fixed percentages per channel. While these methods provide a starting point, they often lack flexibility and precision because:
Static budgets do not adapt to real-time shifts in consumer behavior or market trends.
Human bias and assumptions can misallocate funds to less effective segments.
Limited data analysis restricts the ability to predict complex interactions between channels, time of day, and audience segments.
Slow reaction times mean opportunities can be missed or budgets wasted during market fluctuations.
Machine learning addresses these limitations by continuously learning from new data and recalibrating budgets for maximum efficiency.
How Machine Learning Powers Predictive Budget Allocation
1. Data-Driven Insights
ML models ingest large volumes of data—from campaign performance metrics to user behavior, demographic information, and external factors like seasonality or competitor actions. This comprehensive analysis uncovers hidden trends and actionable insights that humans might overlook.
2. Real-Time Optimization
Unlike traditional models, ML algorithms continuously process incoming data, allowing budget adjustments in real time. For example, if a particular audience segment starts showing higher conversion rates or lower cost per acquisition (CPA), the system can automatically shift more budget toward that segment instantly.
3. Cross-Channel Coordination
Programmatic campaigns often run across multiple platforms and devices. ML models can holistically evaluate each channel’s performance and allocate funds where they deliver the best incremental value, preventing overspend on underperforming channels.
4. Forecasting and Scenario Planning
Advanced predictive models simulate various budget scenarios, estimating outcomes such as clicks, conversions, and revenue. Marketers can then choose the optimal budget distribution aligned with their objectives—whether maximizing reach, minimizing CPA, or boosting brand awareness.
Benefits of Machine Learning in Budget Allocation
a. Maximized ROI
By pinpointing the most lucrative audiences, ad placements, and timing, ML-driven budget allocation significantly improves ROI compared to static budgeting methods.
b. Reduced Wastage
Dynamic allocation ensures that budgets are not wasted on ineffective impressions or channels, preserving ad spend for high-impact opportunities.
c. Agility and Speed
The automated nature of ML enables rapid response to changing market conditions or campaign performance, keeping budgets aligned with real-world dynamics.
d. Scalability
Whether managing dozens or thousands of campaigns, machine learning scales effortlessly, delivering consistent optimization without additional manual effort.
Practical Use Cases in Programmatic Advertising
E-commerce campaigns: ML predicts which products and audience segments will generate the highest sales and allocates budget accordingly, maximizing revenue during peak shopping seasons.
Brand awareness campaigns: Algorithms identify optimal times and channels for broad reach while balancing spend against engagement metrics.
Multi-market campaigns: For global or regional advertisers, ML models tailor budget splits to local audience behaviors and platform preferences.
Cross-device targeting: ML helps shift budgets dynamically between desktop, mobile, and Connected TV based on where users engage most effectively.
Challenges and Considerations
While the advantages of ML-driven budget allocation are clear, marketers should keep these points in mind:
Quality of Data: Machine learning models rely heavily on clean, comprehensive, and timely data. Inaccurate or incomplete data can lead to poor predictions.
Model Transparency: Some ML algorithms operate as “black boxes,” making it difficult to interpret budget decisions. Collaborating with data scientists or vendors who provide explainability is essential.
Integration Complexity: Implementing ML requires integration between data sources, demand-side platforms (DSPs), and marketing tech stacks. Planning and resources are key for success.
Privacy Compliance: Ensuring that data usage aligns with GDPR, CCPA, and other privacy regulations is mandatory when feeding user data into ML systems.
The Future of Predictive Budget Allocation
As machine learning technologies evolve, predictive budget allocation will become even more sophisticated, incorporating:
Multi-touch attribution data for more accurate ROI measurement
Cross-device user journeys to optimize budgets based on complete consumer paths
Natural language processing (NLP) for sentiment analysis and market signals
Integration with AI-powered creative optimization for holistic campaign tuning
In North America, where programmatic adoption is mature, and the MENA region, which is rapidly expanding its digital infrastructure, ML-powered budget allocation will be a key driver of competitive advantage.
Conclusion
Machine learning is transforming programmatic advertising by enabling predictive, data-driven budget allocation that maximizes efficiency, agility, and ROI. By moving beyond static rules and manual guesswork, brands can dynamically optimize their media spend in real time, targeting the right audiences on the right channels at the right moments.
For marketers aiming to stay ahead in the complex programmatic landscape of 2025 and beyond, embracing machine learning for predictive budget allocation isn’t just a luxury—it’s a necessity.
Meta Description:
Discover how machine learning enhances predictive budget allocation in programmatic advertising, driving smarter spend decisions and maximizing ROI in digital campaigns.
SEO Keywords:
Machine learning budget allocation, predictive budget allocation programmatic, programmatic advertising optimization, AI in programmatic, digital ad spend management